A new firm called Anthropic, which was formed by former OpenAI researchers, has the AI community all chock full. The business has funded over $1 billion with a $5 billion pre-product valuation, and Claude, their first product, has sparked a lot of interest in the AI community.
Claude is intended to have conversational abilities similar to OpenAI’s ChatGPT, but with a unique method for minimizing harm and maintaining expressiveness.
Anthropic has devised the Constitutional AI (CAI) training approach to hone Claude into a non-evasive and generally benign assistant. By not requiring any labels for harmfulness in human feedback, CAI outperforms conventional reinforcement learning from human feedback.
The term “constitutional” refers to the notion that, even if they are implicit or concealed, a set of guiding principles must be chosen when creating and implementing a general AI system. The AI assistant’s principles act as a kind of constitution, and they can be taught by providing a concise list of principles.
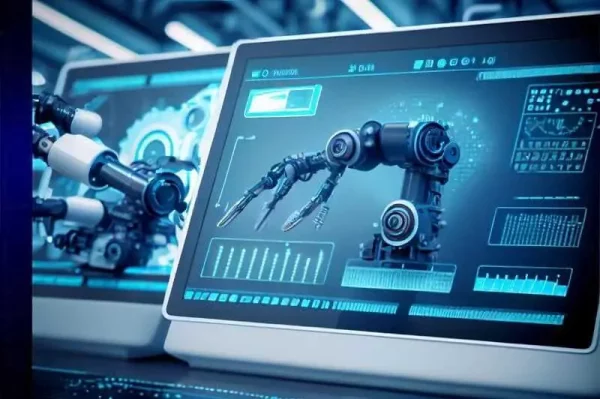
In order to create and implement artificial intelligence systems, AI models must be fine-tuned and trained. This is so because the quality of machine learning algorithms depends on the quality of the training data. As a result, it is essential to train and engineer AI models using substantial, high-quality datasets in an effort to produce accurate and useful results.
The act of modifying pre-trained AI models to match certain use cases is referred to as fine-tuning. This entails changing the model’s parameters to better suit the task at hand, such as translating text between languages or identifying objects in certain photographs.
Compared to models that are trained from scratch, fine-tuning enables AI models to attain higher accuracy and enhanced performance because it makes use of information that has previously been gleaned from sizable, general-purpose datasets.
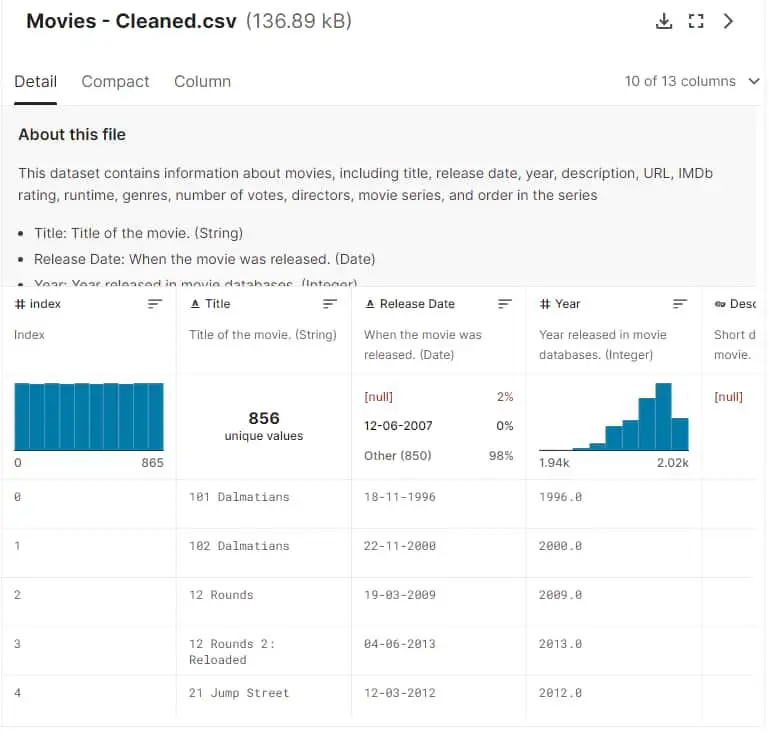
How does the CAI training procedure work?
One of CAI’s four main objectives is to use AI systems to help supervise other AIs and increase supervision scalability. Secondly, to enhance previous work on training a harmless AI assistant by reducing ambiguous responses, easing the tension between helpfulness and harmlessness, and encouraging the AI to explain objections to harmful requests.
Thirdly, it would help increase transparency in the rules governing AI behavior and their application. And lastly, to shorten iteration time by doing away with the requirement to gather additional human input labels each time the goal is changed.
There are two stages to the CAI training procedure. During the first stage, known as supervised learning, an AI assistant that only provides assistance when necessary and creates responses to negative stimuli. In order to fine-tune a pre-trained language model, these responses are scrutinized and altered frequently in accordance with a constitutional principle until they do so.
The second stage of training is comparable to reinforcement learning from human feedback (RLHF), but instead of using human preferences, it makes use of AI feedback (RLAIF).
An AI-human hybrid preference model is trained using a combination of human input for helpfulness and AI-generated preferences for harmlessness. The AI then, assesses replies in accordance with a set of constitutional norms.
Eventually, a policy trained by RLAIF results from the optimization of the language model from the first step using reinforcement learning against the preference model.
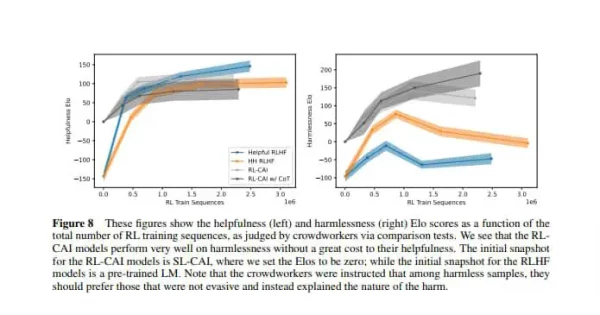
On the other hand, training AI models entails exposing them to large datasets and modifying the model parameters to fit the data. This procedure is performed numerous times until the model has figured out the connection between the input and output data and is able to predict outcomes accurately.
Training is essential for AI models to generalise their understanding to raw, unexplored data. Something which is vital for their practical applications.
To achieve precise and efficient machine learning algorithms, it is necessary to train and fine-tune AI models. These procedures assist AI models in adapting to particular use cases and extrapolating their knowledge to new data, allowing them to produce reliable predictions and judgments.
The relevance of adjusting and training AI models will only expand as the demand for AI rises, making it a crucial area of attention for both researchers and practitioners.
Both chatbots appear to be capable of multi-hop reasoning, despite the fact that Claude’s original version is still in close beta. Claude seems to be even more remarkable in several areas, like the examination of fictional works. The launch of Claude is eagerly awaited and is projected to help advance the field of AI chatbots.